Kubernetes News
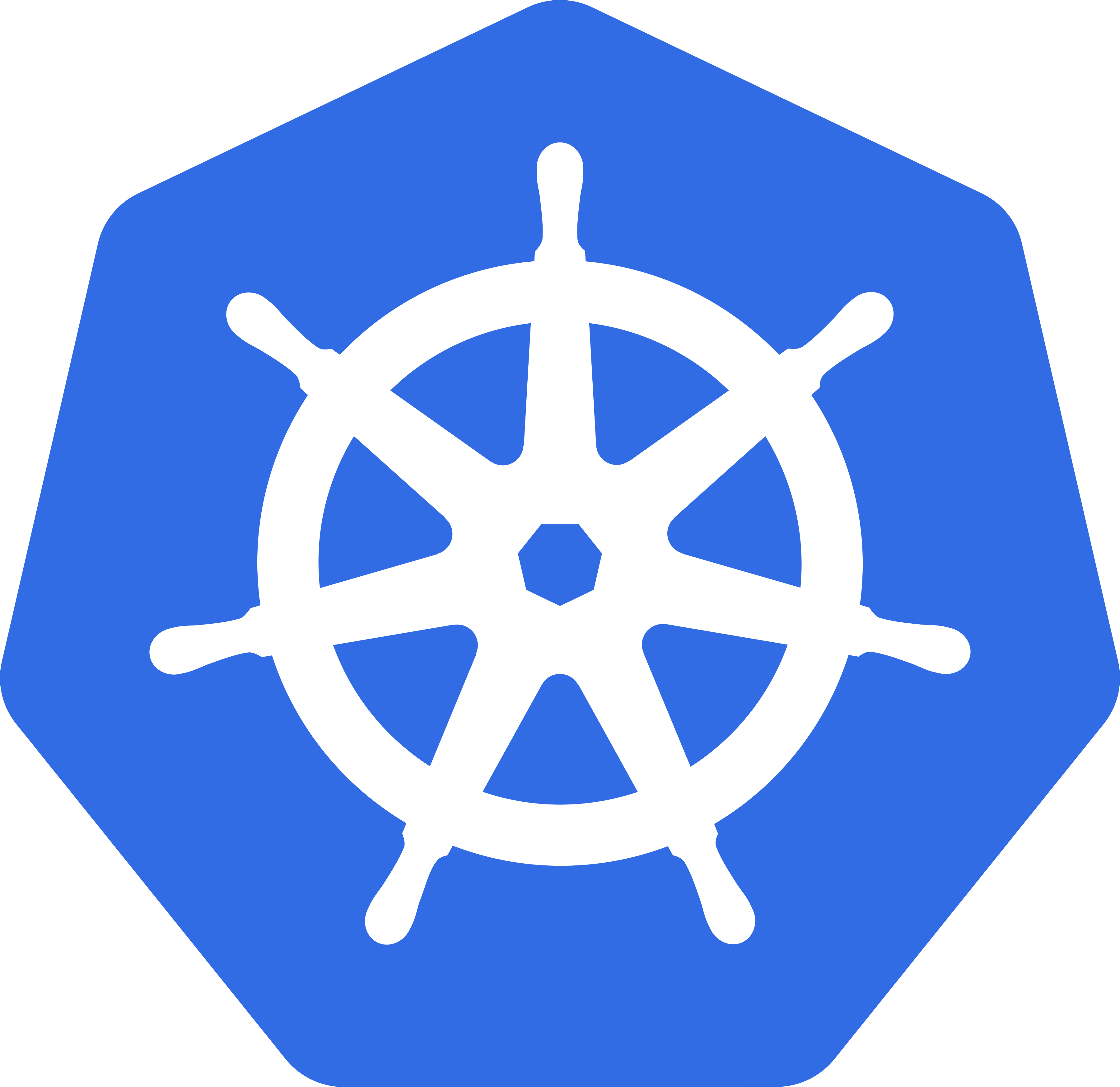
-
Enhancing Kubernetes Event Management with Custom Aggregation
Kubernetes Events provide crucial insights into cluster operations, but as clusters grow, managing and analyzing these events becomes increasingly challenging. This blog post explores how to build custom event aggregation systems that help engineering teams better understand cluster behavior and troubleshoot issues more effectively.
The challenge with Kubernetes events
In a Kubernetes cluster, events are generated for various operations - from pod scheduling and container starts to volume mounts and network configurations. While these events are invaluable for debugging and monitoring, several challenges emerge in production environments:
- Volume: Large clusters can generate thousands of events per minute
- Retention: Default event retention is limited to one hour
- Correlation: Related events from different components are not automatically linked
- Classification: Events lack standardized severity or category classifications
- Aggregation: Similar events are not automatically grouped
To learn more about Events in Kubernetes, read the Event API reference.
Real-World value
Consider a production environment with tens of microservices where the users report intermittent transaction failures:
Traditional event aggregation process: Engineers are wasting hours sifting through thousands of standalone events spread across namespaces. By the time they look into it, the older events have long since purged, and correlating pod restarts to node-level issues is practically impossible.
With its event aggregation in its custom events: The system groups events across resources, instantly surfacing correlation patterns such as volume mount timeouts before pod restarts. History indicates it occurred during past record traffic spikes, highlighting a storage scalability issue in minutes rather than hours.
The benefit of this approach is that organizations that implement it commonly cut down their troubleshooting time significantly along with increasing the reliability of systems by detecting patterns early.
Building an Event aggregation system
This post explores how to build a custom event aggregation system that addresses these challenges, aligned to Kubernetes best practices. I've picked the Go programming language for my example.
Architecture overview
This event aggregation system consists of three main components:
- Event Watcher: Monitors the Kubernetes API for new events
- Event Processor: Processes, categorizes, and correlates events
- Storage Backend: Stores processed events for longer retention
Here's a sketch for how to implement the event watcher:
package main import ( "context" metav1 "k8s.io/apimachinery/pkg/apis/meta/v1" "k8s.io/client-go/kubernetes" "k8s.io/client-go/rest" eventsv1 "k8s.io/api/events/v1" ) type EventWatcher struct { clientset *kubernetes.Clientset } func NewEventWatcher(config *rest.Config) (*EventWatcher, error) { clientset, err := kubernetes.NewForConfig(config) if err != nil { return nil, err } return &EventWatcher{clientset: clientset}, nil } func (w *EventWatcher) Watch(ctx context.Context) (<-chan *eventsv1.Event, error) { events := make(chan *eventsv1.Event) watcher, err := w.clientset.EventsV1().Events("").Watch(ctx, metav1.ListOptions{}) if err != nil { return nil, err } go func() { defer close(events) for { select { case event := <-watcher.ResultChan(): if e, ok := event.Object.(*eventsv1.Event); ok { events <- e } case <-ctx.Done(): watcher.Stop() return } } }() return events, nil }
Event processing and classification
The event processor enriches events with additional context and classification:
type EventProcessor struct { categoryRules []CategoryRule correlationRules []CorrelationRule } type ProcessedEvent struct { Event *eventsv1.Event Category string Severity string CorrelationID string Metadata map[string]string } func (p *EventProcessor) Process(event *eventsv1.Event) *ProcessedEvent { processed := &ProcessedEvent{ Event: event, Metadata: make(map[string]string), } // Apply classification rules processed.Category = p.classifyEvent(event) processed.Severity = p.determineSeverity(event) // Generate correlation ID for related events processed.CorrelationID = p.correlateEvent(event) // Add useful metadata processed.Metadata = p.extractMetadata(event) return processed }
Implementing Event correlation
One of the key features you could implement is a way of correlating related Events. Here's an example correlation strategy:
func (p *EventProcessor) correlateEvent(event *eventsv1.Event) string { // Correlation strategies: // 1. Time-based: Events within a time window // 2. Resource-based: Events affecting the same resource // 3. Causation-based: Events with cause-effect relationships correlationKey := generateCorrelationKey(event) return correlationKey } func generateCorrelationKey(event *eventsv1.Event) string { // Example: Combine namespace, resource type, and name return fmt.Sprintf("%s/%s/%s", event.InvolvedObject.Namespace, event.InvolvedObject.Kind, event.InvolvedObject.Name, ) }
Event storage and retention
For long-term storage and analysis, you'll probably want a backend that supports:
- Efficient querying of large event volumes
- Flexible retention policies
- Support for aggregation queries
Here's a sample storage interface:
type EventStorage interface { Store(context.Context, *ProcessedEvent) error Query(context.Context, EventQuery) ([]ProcessedEvent, error) Aggregate(context.Context, AggregationParams) ([]EventAggregate, error) } type EventQuery struct { TimeRange TimeRange Categories []string Severity []string CorrelationID string Limit int } type AggregationParams struct { GroupBy []string TimeWindow string Metrics []string }
Good practices for Event management
-
Resource Efficiency
- Implement rate limiting for event processing
- Use efficient filtering at the API server level
- Batch events for storage operations
-
Scalability
- Distribute event processing across multiple workers
- Use leader election for coordination
- Implement backoff strategies for API rate limits
-
Reliability
- Handle API server disconnections gracefully
- Buffer events during storage backend unavailability
- Implement retry mechanisms with exponential backoff
Advanced features
Pattern detection
Implement pattern detection to identify recurring issues:
type PatternDetector struct { patterns map[string]*Pattern threshold int } func (d *PatternDetector) Detect(events []ProcessedEvent) []Pattern { // Group similar events groups := groupSimilarEvents(events) // Analyze frequency and timing patterns := identifyPatterns(groups) return patterns } func groupSimilarEvents(events []ProcessedEvent) map[string][]ProcessedEvent { groups := make(map[string][]ProcessedEvent) for _, event := range events { // Create similarity key based on event characteristics similarityKey := fmt.Sprintf("%s:%s:%s", event.Event.Reason, event.Event.InvolvedObject.Kind, event.Event.InvolvedObject.Namespace, ) // Group events with the same key groups[similarityKey] = append(groups[similarityKey], event) } return groups } func identifyPatterns(groups map[string][]ProcessedEvent) []Pattern { var patterns []Pattern for key, events := range groups { // Only consider groups with enough events to form a pattern if len(events) < 3 { continue } // Sort events by time sort.Slice(events, func(i, j int) bool { return events[i].Event.LastTimestamp.Time.Before(events[j].Event.LastTimestamp.Time) }) // Calculate time range and frequency firstSeen := events[0].Event.FirstTimestamp.Time lastSeen := events[len(events)-1].Event.LastTimestamp.Time duration := lastSeen.Sub(firstSeen).Minutes() var frequency float64 if duration > 0 { frequency = float64(len(events)) / duration } // Create a pattern if it meets threshold criteria if frequency > 0.5 { // More than 1 event per 2 minutes pattern := Pattern{ Type: key, Count: len(events), FirstSeen: firstSeen, LastSeen: lastSeen, Frequency: frequency, EventSamples: events[:min(3, len(events))], // Keep up to 3 samples } patterns = append(patterns, pattern) } } return patterns }
With this implementation, the system can identify recurring patterns such as node pressure events, pod scheduling failures, or networking issues that occur with a specific frequency.
Real-time alerts
The following example provides a starting point for building an alerting system based on event patterns. It is not a complete solution but a conceptual sketch to illustrate the approach.
type AlertManager struct { rules []AlertRule notifiers []Notifier } func (a *AlertManager) EvaluateEvents(events []ProcessedEvent) { for _, rule := range a.rules { if rule.Matches(events) { alert := rule.GenerateAlert(events) a.notify(alert) } } }
Conclusion
A well-designed event aggregation system can significantly improve cluster observability and troubleshooting capabilities. By implementing custom event processing, correlation, and storage, operators can better understand cluster behavior and respond to issues more effectively.
The solutions presented here can be extended and customized based on specific requirements while maintaining compatibility with the Kubernetes API and following best practices for scalability and reliability.
Next steps
Future enhancements could include:
- Machine learning for anomaly detection
- Integration with popular observability platforms
- Custom event APIs for application-specific events
- Enhanced visualization and reporting capabilities
For more information on Kubernetes events and custom controllers, refer to the official Kubernetes documentation.
-
Introducing Gateway API Inference Extension
Modern generative AI and large language model (LLM) services create unique traffic-routing challenges on Kubernetes. Unlike typical short-lived, stateless web requests, LLM inference sessions are often long-running, resource-intensive, and partially stateful. For example, a single GPU-backed model server may keep multiple inference sessions active and maintain in-memory token caches.
Traditional load balancers focused on HTTP path or round-robin lack the specialized capabilities needed for these workloads. They also don’t account for model identity or request criticality (e.g., interactive chat vs. batch jobs). Organizations often patch together ad-hoc solutions, but a standardized approach is missing.
Gateway API Inference Extension
Gateway API Inference Extension was created to address this gap by building on the existing Gateway API, adding inference-specific routing capabilities while retaining the familiar model of Gateways and HTTPRoutes. By adding an inference extension to your existing gateway, you effectively transform it into an Inference Gateway, enabling you to self-host GenAI/LLMs with a “model-as-a-service” mindset.
The project’s goal is to improve and standardize routing to inference workloads across the ecosystem. Key objectives include enabling model-aware routing, supporting per-request criticalities, facilitating safe model roll-outs, and optimizing load balancing based on real-time model metrics. By achieving these, the project aims to reduce latency and improve accelerator (GPU) utilization for AI workloads.
How it works
The design introduces two new Custom Resources (CRDs) with distinct responsibilities, each aligning with a specific user persona in the AI/ML serving workflow:
-
InferencePool Defines a pool of pods (model servers) running on shared compute (e.g., GPU nodes). The platform admin can configure how these pods are deployed, scaled, and balanced. An InferencePool ensures consistent resource usage and enforces platform-wide policies. An InferencePool is similar to a Service but specialized for AI/ML serving needs and aware of the model-serving protocol.
-
InferenceModel A user-facing model endpoint managed by AI/ML owners. It maps a public name (e.g., "gpt-4-chat") to the actual model within an InferencePool. This lets workload owners specify which models (and optional fine-tuning) they want served, plus a traffic-splitting or prioritization policy.
In summary, the InferenceModel API lets AI/ML owners manage what is served, while the InferencePool lets platform operators manage where and how it’s served.
Request flow
The flow of a request builds on the Gateway API model (Gateways and HTTPRoutes) with one or more extra inference-aware steps (extensions) in the middle. Here’s a high-level example of the request flow with the Endpoint Selection Extension (ESE):
-
Gateway Routing
A client sends a request (e.g., an HTTP POST to /completions). The Gateway (like Envoy) examines the HTTPRoute and identifies the matching InferencePool backend. -
Endpoint Selection
Instead of simply forwarding to any available pod, the Gateway consults an inference-specific routing extension— the Endpoint Selection Extension—to pick the best of the available pods. This extension examines live pod metrics (queue lengths, memory usage, loaded adapters) to choose the ideal pod for the request. -
Inference-Aware Scheduling
The chosen pod is the one that can handle the request with the lowest latency or highest efficiency, given the user’s criticality or resource needs. The Gateway then forwards traffic to that specific pod.
This extra step provides a smarter, model-aware routing mechanism that still feels like a normal single request to the client. Additionally, the design is extensible—any Inference Gateway can be enhanced with additional inference-specific extensions to handle new routing strategies, advanced scheduling logic, or specialized hardware needs. As the project continues to grow, contributors are encouraged to develop new extensions that are fully compatible with the same underlying Gateway API model, further expanding the possibilities for efficient and intelligent GenAI/LLM routing.
Benchmarks
We evaluated this extension against a standard Kubernetes Service for a vLLM‐based model serving deployment. The test environment consisted of multiple H100 (80 GB) GPU pods running vLLM (version 1) on a Kubernetes cluster, with 10 Llama2 model replicas. The Latency Profile Generator (LPG) tool was used to generate traffic and measure throughput, latency, and other metrics. The ShareGPT dataset served as the workload, and traffic was ramped from 100 Queries per Second (QPS) up to 1000 QPS.
Key results
-
Comparable Throughput: Throughout the tested QPS range, the ESE delivered throughput roughly on par with a standard Kubernetes Service.
-
Lower Latency:
- Per‐Output‐Token Latency: The ESE showed significantly lower p90 latency at higher QPS (500+), indicating that its model-aware routing decisions reduce queueing and resource contention as GPU memory approaches saturation.
- Overall p90 Latency: Similar trends emerged, with the ESE reducing end‐to‐end tail latencies compared to the baseline, particularly as traffic increased beyond 400–500 QPS.
These results suggest that this extension's model‐aware routing significantly reduced latency for GPU‐backed LLM workloads. By dynamically selecting the least‐loaded or best‐performing model server, it avoids hotspots that can appear when using traditional load balancing methods for large, long‐running inference requests.
Roadmap
As the Gateway API Inference Extension heads toward GA, planned features include:
- Prefix-cache aware load balancing for remote caches
- LoRA adapter pipelines for automated rollout
- Fairness and priority between workloads in the same criticality band
- HPA support for scaling based on aggregate, per-model metrics
- Support for large multi-modal inputs/outputs
- Additional model types (e.g., diffusion models)
- Heterogeneous accelerators (serving on multiple accelerator types with latency- and cost-aware load balancing)
- Disaggregated serving for independently scaling pools
Summary
By aligning model serving with Kubernetes-native tooling, Gateway API Inference Extension aims to simplify and standardize how AI/ML traffic is routed. With model-aware routing, criticality-based prioritization, and more, it helps ops teams deliver the right LLM services to the right users—smoothly and efficiently.
Ready to learn more? Visit the project docs to dive deeper, give an Inference Gateway extension a try with a few simple steps, and get involved if you’re interested in contributing to the project!
-
-
Start Sidecar First: How To Avoid Snags
From the Kubernetes Multicontainer Pods: An Overview blog post you know what their job is, what are the main architectural patterns, and how they are implemented in Kubernetes. The main thing I’ll cover in this article is how to ensure that your sidecar containers start before the main app. It’s more complicated than you might think!
A gentle refresher
I'd just like to remind readers that the v1.29.0 release of Kubernetes added native support for sidecar containers, which can now be defined within the
.spec.initContainers
field, but withrestartPolicy: Always
. You can see that illustrated in the following example Pod manifest snippet:initContainers: - name:logshipper image:alpine:latest restartPolicy:Always# this is what makes it a sidecar container command:['sh','-c','tail -F /opt/logs.txt'] volumeMounts: - name:data mountPath:/opt
What are the specifics of defining sidecars with a
.spec.initContainers
block, rather than as a legacy multi-container pod with multiple.spec.containers
? Well, all.spec.initContainers
are always launched before the main application. If you define Kubernetes-native sidecars, those are terminated after the main application. Furthermore, when used with Jobs, a sidecar container should still be alive and could potentially even restart after the owning Job is complete; Kubernetes-native sidecar containers do not block pod completion.To learn more, you can also read the official Pod sidecar containers tutorial.
The problem
Now you know that defining a sidecar with this native approach will always start it before the main application. From the kubelet source code, it's visible that this often means being started almost in parallel, and this is not always what an engineer wants to achieve. What I'm really interested in is whether I can delay the start of the main application until the sidecar is not just started, but fully running and ready to serve. It might be a bit tricky because the problem with sidecars is there’s no obvious success signal, contrary to init containers - designed to run only for a specified period of time. With an init container, exit status 0 is unambiguously "I succeeded". With a sidecar, there are lots of points at which you can say "a thing is running". Starting one container only after the previous one is ready is part of a graceful deployment strategy, ensuring proper sequencing and stability during startup. It’s also actually how I’d expect sidecar containers to work as well, to cover the scenario where the main application is dependent on the sidecar. For example, it may happen that an app errors out if the sidecar isn’t available to serve requests (e.g., logging with DataDog). Sure, one could change the application code (and it would actually be the “best practice” solution), but sometimes they can’t - and this post focuses on this use case.
I'll explain some ways that you might try, and show you what approaches will really work.
Readiness probe
To check whether Kubernetes native sidecar delays the start of the main application until the sidecar is ready, let’s simulate a short investigation. Firstly, I’ll simulate a sidecar container which will never be ready by implementing a readiness probe which will never succeed. As a reminder, a readiness probe checks if the container is ready to start accepting traffic and therefore, if the pod can be used as a backend for services.
(Unlike standard init containers, sidecar containers can have probes so that the kubelet can supervise the sidecar and intervene if there are problems. For example, restarting a sidecar container if it fails a health check.)
apiVersion:apps/v1 kind:Deployment metadata: name:myapp labels: app:myapp spec: replicas:1 selector: matchLabels: app:myapp template: metadata: labels: app:myapp spec: containers: - name:myapp image:alpine:latest command:["sh","-c","sleep 3600"] initContainers: - name:nginx image:nginx:latest restartPolicy:Always ports: - containerPort:80 protocol:TCP readinessProbe: exec: command: - /bin/sh - -c - exit 1# this command always fails, keeping the container "Not Ready" periodSeconds:5 volumes: - name:data emptyDir:{}
The result is:
controlplane $ kubectl get pods -w NAME READY STATUS RESTARTS AGE myapp-db5474f45-htgw5 1/2 Running 0 9m28s controlplane $ kubectl describe pod myapp-db5474f45-htgw5 Name: myapp-db5474f45-htgw5 Namespace: default (...) Events: Type Reason Age From Message ---- ------ ---- ---- ------- Normal Scheduled 17s default-scheduler Successfully assigned default/myapp-db5474f45-htgw5 to node01 Normal Pulling 16s kubelet Pulling image "nginx:latest" Normal Pulled 16s kubelet Successfully pulled image "nginx:latest" in 163ms (163ms including waiting). Image size: 72080558 bytes. Normal Created 16s kubelet Created container nginx Normal Started 16s kubelet Started container nginx Normal Pulling 15s kubelet Pulling image "alpine:latest" Normal Pulled 15s kubelet Successfully pulled image "alpine:latest" in 159ms (160ms including waiting). Image size: 3652536 bytes. Normal Created 15s kubelet Created container myapp Normal Started 15s kubelet Started container myapp Warning Unhealthy 1s (x6 over 15s) kubelet Readiness probe failed:
From these logs it’s evident that only one container is ready - and I know it can’t be the sidecar, because I’ve defined it so it’ll never be ready (you can also check container statuses in
kubectl get pod -o json
). I also saw that myapp has been started before the sidecar is ready. That was not the result I wanted to achieve; in this case, the main app container has a hard dependency on its sidecar.Maybe a startup probe?
To ensure that the sidecar is ready before the main app container starts, I can define a
startupProbe
. It will delay the start of the main container until the command is successfully executed (returns0
exit status). If you’re wondering why I’ve added it to myinitContainer
, let’s analyse what happens If I’d added it to myapp container. I wouldn’t have guaranteed the probe would run before the main application code - and this one, can potentially error out without the sidecar being up and running.apiVersion:apps/v1 kind:Deployment metadata: name:myapp labels: app:myapp spec: replicas:1 selector: matchLabels: app:myapp template: metadata: labels: app:myapp spec: containers: - name:myapp image:alpine:latest command:["sh","-c","sleep 3600"] initContainers: - name:nginx image:nginx:latest ports: - containerPort:80 protocol:TCP restartPolicy:Always startupProbe: httpGet: path:/ port:80 initialDelaySeconds:5 periodSeconds:30 failureThreshold:10 timeoutSeconds:20 volumes: - name:data emptyDir:{}
This results in 2/2 containers being ready and running, and from events, it can be inferred that the main application started only after nginx had already been started. But to confirm whether it waited for the sidecar readiness, let’s change the
startupProbe
to the exec type of command:startupProbe: exec: command: - /bin/sh - -c - sleep 15
and run
kubectl get pods -w
to watch in real time whether the readiness of both containers only changes after a 15 second delay. Again, events confirm the main application starts after the sidecar. That means that using thestartupProbe
with a correctstartupProbe.httpGet
request helps to delay the main application start until the sidecar is ready. It’s not optimal, but it works.What about the postStart lifecycle hook?
Fun fact: using the
postStart
lifecycle hook block will also do the job, but I’d have to write my own mini-shell script, which is even less efficient.initContainers: - name:nginx image:nginx:latest restartPolicy:Always ports: - containerPort:80 protocol:TCP lifecycle: postStart: exec: command: - /bin/sh - -c - | echo "Waiting for readiness at http://localhost:80" until curl -sf http://localhost:80; do echo "Still waiting for http://localhost:80..." sleep 5 done echo "Service is ready at http://localhost:80"
Liveness probe
An interesting exercise would be to check the sidecar container behavior with a liveness probe. A liveness probe behaves and is configured similarly to a readiness probe - only with the difference that it doesn’t affect the readiness of the container but restarts it in case the probe fails.
livenessProbe: exec: command: - /bin/sh - -c - exit 1# this command always fails, keeping the container "Not Ready" periodSeconds:5
After adding the liveness probe configured just as the previous readiness probe and checking events of the pod by
kubectl describe pod
it’s visible that the sidecar has a restart count above 0. Nevertheless, the main application is not restarted nor influenced at all, even though I'm aware that (in our imaginary worst-case scenario) it can error out when the sidecar is not there serving requests. What if I’d used alivenessProbe
without lifecyclepostStart
? Both containers will be immediately ready: at the beginning, this behavior will not be different from the one without any additional probes since the liveness probe doesn’t affect readiness at all. After a while, the sidecar will begin to restart itself, but it won’t influence the main container.Findings summary
I’ll summarize the startup behavior in the table below:
Probe/Hook Sidecar starts before the main app? Main app waits for the sidecar to be ready? What if the check doesn’t pass? readinessProbe
Yes, but it’s almost in parallel (effectively no) No Sidecar is not ready; main app continues running livenessProbe
Yes, but it’s almost in parallel (effectively no) No Sidecar is restarted, main app continues running startupProbe
Yes Yes Main app is not started postStart Yes, main app container starts after postStart
completesYes, but you have to provide custom logic for that Main app is not started To summarize: with sidecars often being a dependency of the main application, you may want to delay the start of the latter until the sidecar is healthy. The ideal pattern is to start both containers simultaneously and have the app container logic delay at all levels, but it’s not always possible. If that's what you need, you have to use the right kind of customization to the Pod definition. Thankfully, it’s nice and quick, and you have the recipe ready above.
Happy deploying!
-
Gateway API v1.3.0: Advancements in Request Mirroring, CORS, Gateway Merging, and Retry Budgets
Join us in the Kubernetes SIG Network community in celebrating the general availability of Gateway API v1.3.0! We are also pleased to announce that there are already a number of conformant implementations to try, made possible by postponing this blog announcement. Version 1.3.0 of the API was released about a month ago on April 24, 2025.
Gateway API v1.3.0 brings a new feature to the Standard channel (Gateway API's GA release channel): percentage-based request mirroring, and introduces three new experimental features: cross-origin resource sharing (CORS) filters, a standardized mechanism for listener and gateway merging, and retry budgets.
Also see the full release notes and applaud the v1.3.0 release team next time you see them.
Graduation to Standard channel
Graduation to the Standard channel is a notable achievement for Gateway API features, as inclusion in the Standard release channel denotes a high level of confidence in the API surface and provides guarantees of backward compatibility. Of course, as with any other Kubernetes API, Standard channel features can continue to evolve with backward-compatible additions over time, and we (SIG Network) certainly expect further refinements and improvements in the future. For more information on how all of this works, refer to the Gateway API Versioning Policy.
Percentage-based request mirroring
Leads: Lior Lieberman,Jake Bennert
GEP-3171: Percentage-Based Request Mirroring
Percentage-based request mirroring is an enhancement to the existing support for HTTP request mirroring, which allows HTTP requests to be duplicated to another backend using the RequestMirror filter type. Request mirroring is particularly useful in blue-green deployment. It can be used to assess the impact of request scaling on application performance without impacting responses to clients.
The previous mirroring capability worked on all the requests to a
backendRef
.
Percentage-based request mirroring allows users to specify a subset of requests they want to be mirrored, either by percentage or fraction. This can be particularly useful when services are receiving a large volume of requests. Instead of mirroring all of those requests, this new feature can be used to mirror a smaller subset of them.Here's an example with 42% of the requests to "foo-v1" being mirrored to "foo-v2":
apiVersion:gateway.networking.k8s.io/v1 kind:HTTPRoute metadata: name:http-filter-mirror labels: gateway:mirror-gateway spec: parentRefs: - name:mirror-gateway hostnames: - mirror.example rules: - backendRefs: - name:foo-v1 port:8080 filters: - type:RequestMirror requestMirror: backendRef: name:foo-v2 port:8080 percent:42# This value must be an integer.
You can also configure the partial mirroring using a fraction. Here is an example with 5 out of every 1000 requests to "foo-v1" being mirrored to "foo-v2".
rules: - backendRefs: - name:foo-v1 port:8080 filters: - type:RequestMirror requestMirror: backendRef: name:foo-v2 port:8080 fraction: numerator:5 denominator:1000
Additions to Experimental channel
The Experimental channel is Gateway API's channel for experimenting with new features and gaining confidence with them before allowing them to graduate to standard. Please note: the experimental channel may include features that are changed or removed later.
Starting in release v1.3.0, in an effort to distinguish Experimental channel resources from Standard channel resources, any new experimental API kinds have the prefix "X". For the same reason, experimental resources are now added to the API group
gateway.networking.x-k8s.io
instead ofgateway.networking.k8s.io
. Bear in mind that using new experimental channel resources means they can coexist with standard channel resources, but migrating these resources to the standard channel will require recreating them with the standard channel names and API group (both of which lack the "x-k8s" designator or "X" prefix).The v1.3 release introduces two new experimental API kinds: XBackendTrafficPolicy and XListenerSet. To be able to use experimental API kinds, you need to install the Experimental channel Gateway API YAMLs from the locations listed below.
CORS filtering
Leads: Liang Li, Eyal Pazz, Rob Scott
GEP-1767: CORS Filter
Cross-origin resource sharing (CORS) is an HTTP-header based mechanism that allows a web page to access restricted resources from a server on an origin (domain, scheme, or port) different from the domain that served the web page. This feature adds a new HTTPRoute
filter
type, called "CORS", to configure the handling of cross-origin requests before the response is sent back to the client.To be able to use experimental CORS filtering, you need to install the Experimental channel Gateway API HTTPRoute yaml.
Here's an example of a simple cross-origin configuration:
apiVersion:gateway.networking.k8s.io/v1 kind:HTTPRoute metadata: name:http-route-cors spec: parentRefs: - name:http-gateway rules: - matches: - path: type:PathPrefix value:/resource/foo filters: - cors: - type:CORS allowOrigins: - * allowMethods: - GET - HEAD - POST allowHeaders: - Accept - Accept-Language - Content-Language - Content-Type - Range backendRefs: - kind:Service name:http-route-cors port:80
In this case, the Gateway returns an origin header of "*", which means that the requested resource can be referenced from any origin, a methods header (
Access-Control-Allow-Methods
) that permits theGET
,HEAD
, andPOST
verbs, and a headers header allowingAccept
,Accept-Language
,Content-Language
,Content-Type
, andRange
.HTTP/1.1 200 OK Access-Control-Allow-Origin: * Access-Control-Allow-Methods: GET, HEAD, POST Access-Control-Allow-Headers: Accept,Accept-Language,Content-Language,Content-Type,Range
The complete list of fields in the new CORS filter:
allowOrigins
allowMethods
allowHeaders
allowCredentials
exposeHeaders
maxAge
See CORS protocol for details.
XListenerSets (standardized mechanism for Listener and Gateway merging)
Lead: Dave Protasowski
GEP-1713: ListenerSets - Standard Mechanism to Merge Multiple Gateways
This release adds a new experimental API kind, XListenerSet, that allows a shared list of listeners to be attached to one or more parent Gateway(s). In addition, it expands upon the existing suggestion that Gateway API implementations may merge configuration from multiple Gateway objects. It also:
- adds a new field
allowedListeners
to the.spec
of a Gateway. TheallowedListeners
field defines from which Namespaces to select XListenerSets that are allowed to attach to that Gateway: Same, All, None, or Selector based. - increases the previous maximum number (64) of listeners with the addition of XListenerSets.
- allows the delegation of listener configuration, such as TLS, to applications in other namespaces.
To be able to use experimental XListenerSet, you need to install the Experimental channel Gateway API XListenerSet yaml.
The following example shows a Gateway with an HTTP listener and two child HTTPS XListenerSets with unique hostnames and certificates. The combined set of listeners attached to the Gateway includes the two additional HTTPS listeners in the XListenerSets that attach to the Gateway. This example illustrates the delegation of listener TLS config to application owners in different namespaces ("store" and "app"). The HTTPRoute has both the Gateway listener named "foo" and one XListenerSet listener named "second" as
parentRefs
.apiVersion:gateway.networking.k8s.io/v1 kind:Gateway metadata: name:prod-external namespace:infra spec: gatewayClassName:example allowedListeners: - from:All listeners: - name:foo hostname:foo.com protocol:HTTP port:80 --- apiVersion:gateway.networking.x-k8s.io/v1alpha1 kind:XListenerSet metadata: name:store namespace:store spec: parentRef: name:prod-external listeners: - name:first hostname:first.foo.com protocol:HTTPS port:443 tls: mode:Terminate certificateRefs: - kind:Secret group:"" name:first-workload-cert --- apiVersion:gateway.networking.x-k8s.io/v1alpha1 kind:XListenerSet metadata: name:app namespace:app spec: parentRef: name:prod-external listeners: - name:second hostname:second.foo.com protocol:HTTPS port:443 tls: mode:Terminate certificateRefs: - kind:Secret group:"" name:second-workload-cert --- apiVersion:gateway.networking.k8s.io/v1 kind:HTTPRoute metadata: name:httproute-example spec: parentRefs: - name:app kind:XListenerSet sectionName:second - name:parent-gateway kind:Gateway sectionName:foo ...
Each listener in a Gateway must have a unique combination of
port
,protocol
, (andhostname
if supported by the protocol) in order for all listeners to be compatible and not conflicted over which traffic they should receive.Furthermore, implementations can merge separate Gateways into a single set of listener addresses if all listeners across those Gateways are compatible. The management of merged listeners was under-specified in releases prior to v1.3.0.
With the new feature, the specification on merging is expanded. Implementations must treat the parent Gateways as having the merged list of all listeners from itself and from attached XListenerSets, and validation of this list of listeners must behave the same as if the list were part of a single Gateway. Within a single Gateway, listeners are ordered using the following precedence:
- Single Listeners (not a part of an XListenerSet) first,
- Remaining listeners ordered by:
- object creation time (oldest first), and if two listeners are defined in objects that have the same timestamp, then
- alphabetically based on "{namespace}/{name of listener}"
Retry budgets (XBackendTrafficPolicy)
Leads: Eric Bishop, Mike Morris
GEP-3388: Retry Budgets
This feature allows you to configure a retry budget across all endpoints of a destination Service. This is used to limit additional client-side retries after reaching a configured threshold. When configuring the budget, the maximum percentage of active requests that may consist of retries may be specified, as well as the interval over which requests will be considered when calculating the threshold for retries. The development of this specification changed the existing experimental API kind BackendLBPolicy into a new experimental API kind, XBackendTrafficPolicy, in the interest of reducing the proliferation of policy resources that had commonalities.
To be able to use experimental retry budgets, you need to install the Experimental channel Gateway API XBackendTrafficPolicy yaml.
The following example shows an XBackendTrafficPolicy that applies a
retryConstraint
that represents a budget that limits the retries to a maximum of 20% of requests, over a duration of 10 seconds, and to a minimum of 3 retries over 1 second.apiVersion:gateway.networking.x-k8s.io/v1alpha1 kind:XBackendTrafficPolicy metadata: name:traffic-policy-example spec: retryConstraint: budget: percent:20 interval:10s minRetryRate: count:3 interval:1s ...
Try it out
Unlike other Kubernetes APIs, you don't need to upgrade to the latest version of Kubernetes to get the latest version of Gateway API. As long as you're running Kubernetes 1.26 or later, you'll be able to get up and running with this version of Gateway API.
To try out the API, follow the Getting Started Guide. As of this writing, four implementations are already conformant with Gateway API v1.3 experimental channel features. In alphabetical order:
Get involved
Wondering when a feature will be added? There are lots of opportunities to get involved and help define the future of Kubernetes routing APIs for both ingress and service mesh.
- Check out the user guides to see what use-cases can be addressed.
- Try out one of the existing Gateway controllers.
- Or join us in the community and help us build the future of Gateway API together!
The maintainers would like to thank everyone who's contributed to Gateway API, whether in the form of commits to the repo, discussion, ideas, or general support. We could never have made this kind of progress without the support of this dedicated and active community.
Related Kubernetes blog articles
- Gateway API v1.2: WebSockets, Timeouts, Retries, and More (November 2024)
- Gateway API v1.1: Service mesh, GRPCRoute, and a whole lot more (May 2024)
- New Experimental Features in Gateway API v1.0 (November 2023)
- Gateway API v1.0: GA Release (October 2023)
-
Kubernetes v1.33: In-Place Pod Resize Graduated to Beta
On behalf of the Kubernetes project, I am excited to announce that the in-place Pod resize feature (also known as In-Place Pod Vertical Scaling), first introduced as alpha in Kubernetes v1.27, has graduated to Beta and will be enabled by default in the Kubernetes v1.33 release! This marks a significant milestone in making resource management for Kubernetes workloads more flexible and less disruptive.
What is in-place Pod resize?
Traditionally, changing the CPU or memory resources allocated to a container required restarting the Pod. While acceptable for many stateless applications, this could be disruptive for stateful services, batch jobs, or any workloads sensitive to restarts.
In-place Pod resizing allows you to change the CPU and memory requests and limits assigned to containers within a running Pod, often without requiring a container restart.
Here's the core idea:
- The
spec.containers[*].resources
field in a Pod specification now represents the desired resources and is mutable for CPU and memory. - The
status.containerStatuses[*].resources
field reflects the actual resources currently configured on a running container. - You can trigger a resize by updating the desired resources in the Pod spec via the new
resize
subresource.
You can try it out on a v1.33 Kubernetes cluster by using kubectl to edit a Pod (requires
kubectl
v1.32+):kubectl edit pod <pod-name> --subresource resize
For detailed usage instructions and examples, please refer to the official Kubernetes documentation: Resize CPU and Memory Resources assigned to Containers.
Why does in-place Pod resize matter?
Kubernetes still excels at scaling workloads horizontally (adding or removing replicas), but in-place Pod resizing unlocks several key benefits for vertical scaling:
- Reduced Disruption: Stateful applications, long-running batch jobs, and sensitive workloads can have their resources adjusted without suffering the downtime or state loss associated with a Pod restart.
- Improved Resource Utilization: Scale down over-provisioned Pods without disruption, freeing up resources in the cluster. Conversely, provide more resources to Pods under heavy load without needing a restart.
- Faster Scaling: Address transient resource needs more quickly. For example Java applications often need more CPU during startup than during steady-state operation. Start with higher CPU and resize down later.
What's changed between Alpha and Beta?
Since the alpha release in v1.27, significant work has gone into maturing the feature, improving its stability, and refining the user experience based on feedback and further development. Here are the key changes:
Notable user-facing changes
resize
Subresource: Modifying Pod resources must now be done via the Pod'sresize
subresource (kubectl patch pod <name> --subresource resize...
).kubectl
versions v1.32+ support this argument.- Resize Status via Conditions: The old
status.resize
field is deprecated. The status of a resize operation is now exposed via two Pod conditions:PodResizePending
: Indicates the Kubelet cannot grant the resize immediately (e.g.,reason: Deferred
if temporarily unable,reason: Infeasible
if impossible on the node).PodResizeInProgress
: Indicates the resize is accepted and being applied. Errors encountered during this phase are now reported in this condition's message withreason: Error
.
- Sidecar Support: Resizing sidecar containers in-place is now supported.
Stability and reliability enhancements
- Refined Allocated Resources Management: The allocation management logic with the Kubelet was significantly reworked, making it more consistent and robust. The changes eliminated whole classes of bugs, and greatly improved the reliability of in-place Pod resize.
- Improved Checkpointing & State Tracking: A more robust system for tracking "allocated" and "actuated" resources was implemented, using new checkpoint files (
allocated_pods_state
,actuated_pods_state
) to reliably manage resize state across Kubelet restarts and handle edge cases where runtime-reported resources differ from requested ones. Several bugs related to checkpointing and state restoration were fixed. Checkpointing efficiency was also improved. - Faster Resize Detection: Enhancements to the Kubelet's Pod Lifecycle Event Generator (PLEG) allow the Kubelet to respond to and complete resizes much more quickly.
- Enhanced CRI Integration: A new
UpdatePodSandboxResources
CRI call was added to better inform runtimes and plugins (like NRI) about Pod-level resource changes. - Numerous Bug Fixes: Addressed issues related to systemd cgroup drivers, handling of containers without limits, CPU minimum share calculations, container restart backoffs, error propagation, test stability, and more.
What's next?
Graduating to Beta means the feature is ready for broader adoption, but development doesn't stop here! Here's what the community is focusing on next:
- Stability and Productionization: Continued focus on hardening the feature, improving performance, and ensuring it is robust for production environments.
- Addressing Limitations: Working towards relaxing some of the current limitations noted in the documentation, such as allowing memory limit decreases.
- VerticalPodAutoscaler (VPA) Integration: Work to enable VPA to leverage in-place Pod resize is already underway. A new
InPlaceOrRecreate
update mode will allow it to attempt non-disruptive resizes first, or fall back to recreation if needed. This will allow users to benefit from VPA's recommendations with significantly less disruption. - User Feedback: Gathering feedback from users adopting the beta feature is crucial for prioritizing further enhancements and addressing any uncovered issues or bugs.
Getting started and providing feedback
With the
InPlacePodVerticalScaling
feature gate enabled by default in v1.33, you can start experimenting with in-place Pod resizing right away!Refer to the documentation for detailed guides and examples.
As this feature moves through Beta, your feedback is invaluable. Please report any issues or share your experiences via the standard Kubernetes communication channels (GitHub issues, mailing lists, Slack). You can also review the KEP-1287: In-place Update of Pod Resources for the full in-depth design details.
We look forward to seeing how the community leverages in-place Pod resize to build more efficient and resilient applications on Kubernetes!
- The